Self-driving cars are coming. But for all their fancy sensors and intricate data-crunching abilities, even the most cutting-edge cars lack something that (almost) every 16-year-old with a learner’s permit has: social awareness. While autonomous technologies have improved substantially, they still ultimately view the drivers around them as obstacles made up of ones and zeros, rather than human beings with specific intentions, motivations and personalities.
Recently a team of researchers from TU Delft and the MIT’s Computer Science and Artificial Intelligence Laboratory (CSAIL), have been exploring whether self-driving cars can be programmed to classify the social personalities of other drivers, so that they can better predict what different cars will do – and, therefore, be able to drive more safely among them. In the new publication in PNAS, the scientists have integrated tools from social psychology to classify driving behavior with respect to how selfish or selfless a particular driver is.
‘This work enables an autonomous vehicle to reason about the intentions of other traffic participants and the consequences of its own actions.’
dr. Javier Alonso-Mora, TU Delft
TU Delft researcher dr. Javier Alonso-Mora (formerly at MIT) and one of the authors of the publication, explains: ‘This work enables an autonomous vehicle to reason about the intentions of other traffic participants and the consequences of its own actions.’ The researchers specifically use something called Social Value Orientation (SVO), which represents the degree to which someone is selfish (“egoistic”) versus altruistic or cooperative (“prosocial”). The system then estimates drivers’ SVOs to create real-time driving trajectories for self-driving cars. Alonso-Mora: ‘The use of SVO in a game theoretical motion planning framework will not only make autonomous vehicles smarter but it will also lead to improvements in how robots interact with other robots and humans.’
Better predictions
Testing their algorithm on the tasks of merging lanes and making unprotected left turns, the team showed that they could better predict the behaviour of other cars by a factor of 25 percent. For example, the car knew to wait to make a left turn when the approaching car had a more egoistic driver, but make the more aggressive left turn when the other car is “prosocial.”
While not yet robust enough to be implemented on real roads, the system could have some intriguing use cases, and not just for the cars that drive themselves. Say you’re driving along and a car suddenly enters your blind spot – the system could give you a warning in the rearview mirror that the car has an aggressive driver, allowing you to adjust accordingly. It could also allow self-driving cars to actually learn to exhibit more human-like behavior that will be easier for human drivers to understand.
‘Working with and around humans means figuring out their intentions to better understand their behaviour’, says MIT graduate student Wilko Schwarting, who was lead author of the PNAS paper. ‘People’s tendencies to be collaborative or competitive often spills over into how they behave as drivers. In this paper we sought to understand if this was something we could actually quantify.’
‘Working with and around humans means figuring out their intentions to better understand their behaviour’
Wilko Schwarting, MIT graduate student
All the same
A central issue with today’s self-driving cars is that they’re programmed to assume that all humans act the same way. This means that, among other things, they’re quite conservative in their decision-making at four-way stops and other intersections. While this caution reduces the chance of fatal accidents, it also creates bottlenecks that can be frustrating for other drivers, not to mention hard for them to understand. This may be why the majority of traffic incidents have involved getting rear-ended by impatient drivers.
‘Creating more human-like behavior in autonomous vehicles (AVs) is fundamental for the safety of passengers and surrounding vehicles, since behaving in a predictable manner enables humans to understand and appropriately respond to the AV’s actions’, says Schwarting.
To try to expand the car’s social awareness, the CSAIL team combined methods from social psychology with game theory, a theoretical framework for conceiving social situations among competing players. The team modeled road scenarios where each driver tried to maximize their own utility and analyzed their “best responses” given the decisions of all other agents. Based on that small snippet of motion from other cars, the team’s algorithm could then predict the surrounding cars’ behavior as cooperative, altruistic, or egoistic — grouping the first two as “prosocial.” People’s scores for these qualities rest on a continuum with respect to how much a person demonstrates care for themselves versus care for others.
‘This work opens the door to safer and more seamless road-sharing between human-driven and robot-driven cars’
Daniela Rus, MIT
In the merging and left-turn scenarios, the two outcome options were to either let somebody merge into your lane (“prosocial”) or not (“egoistic”). The team’s results showed that, not surprisingly, merging cars are more competitive than non-merging cars. The system was trained to try to better navigate when it’s appropriate to exhibit different behaviors. For example, even the most deferential of human drivers knows that certain types of actions – like making a lane change in heavy traffic – require a moment of being more assertive and decisive.
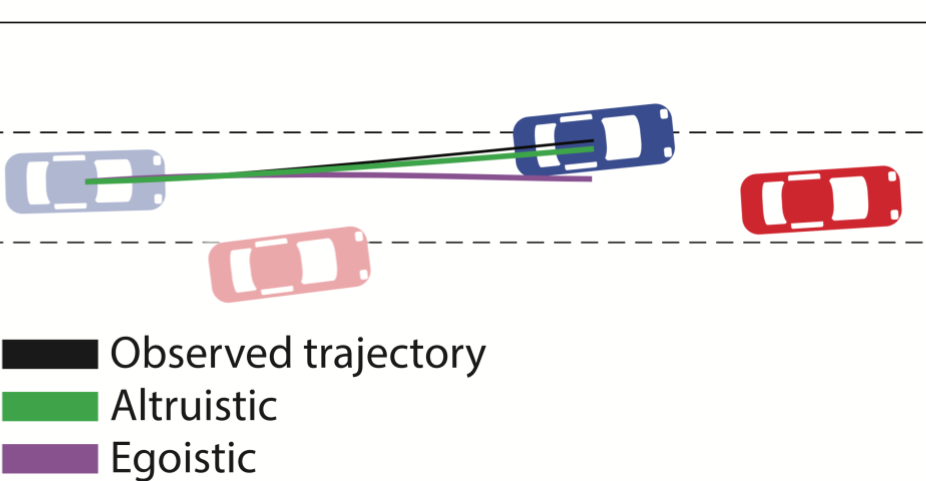
Next phase
For the next phase of the research, the team plans to work to apply their model to pedestrians, bicycles and other agents in driving environments. In addition, they will be investigating other robotic systems acting among humans, such as household robots, and integrating SVO into their prediction and decision-making algorithms. Alyssa Pierson, co-author and research scientist at MIT CSAIL, says that the ability to estimate SVO distributions directly from observed motion instead of in laboratory conditions will be important for fields far beyond autonomous driving.
‘By modeling driving personalities and incorporating the models into the decision making modules of robot cars using the SVO, this work opens the door to safer and more seamless road-sharing between human-driven and robot-driven cars’, says co-author Daniela Rus from MIT.
Click here to read the full press release.